Be the Beat
What if dance leads music? 2024.
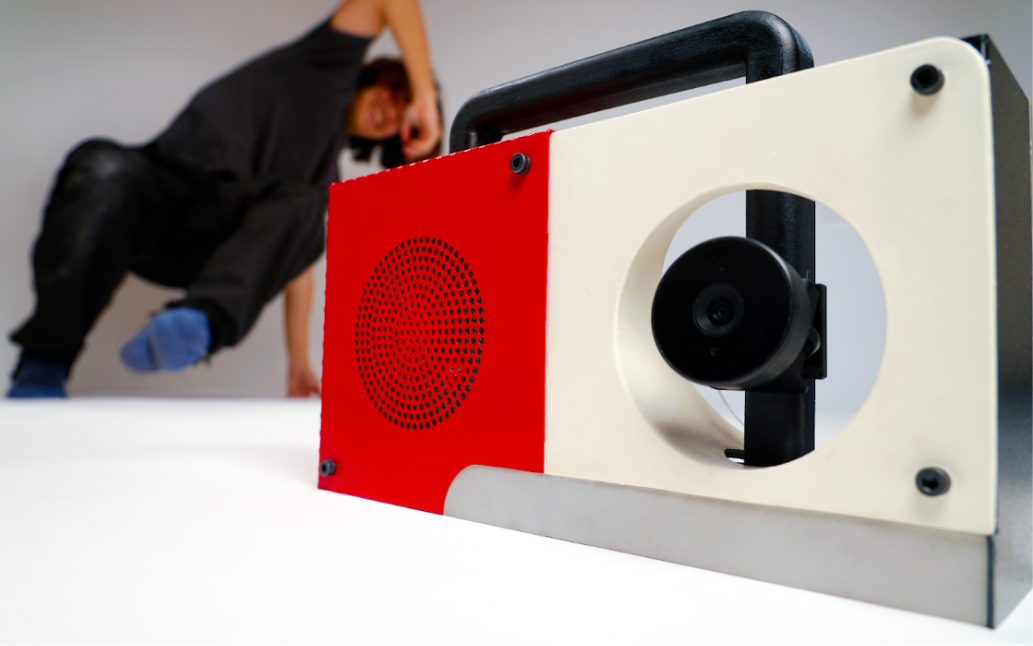
AI-Powered Boombox for Music Generation from Freestyle Dance
Dance has traditionally been guided by music throughout history and across cultures, yet the concept of dancing to create music
is rarely explored. Be the Beat is an AI-powered boombox designed to generate music from a dancer's
movement. Be the Beat uses PoseNet to describe movements for a large language model, enabling it to analyze dance style and query
APIs to find music with similar style, energy, and tempo. In our pilot trials, the boombox successfully matched music to the tempo of
the dancer's movements and even distinguished the intricacies between house and Hip-Hop moves
Collaborator: Zhixing Chen
2024 Neurips Creative AI Track
2025 TEI Work in Progress
Project Description
Be the Beat transforms dance movements into music through AI technologies, creating a unique, interactive experience.
The device can be used when dancers have a specific feeling of dance moves in their mind but can't come up with a
song. Triggered by a hand clap from the user, this activates the workflow below:
- Dancer wants to find a song that fits their freestyle inspiration in the moment but doesn't have a specific song in mind.
- Dancer turns on Be the Beat and claps to start the recording process.
- Be the Beat captures live poses in the dancer's performance and processes the data.
- Within 5 seconds, the boombox generates a list of recommended songs from Spotify based on the dance features and starts playing the first song.
- Dance hears the song played by the speaker and starts dancing to the selected song.
- If the dancer wants another song or shift dance style in certain directions, the dancer can clap and restart the generation process to get new recommendations as their freestyle dance develops.
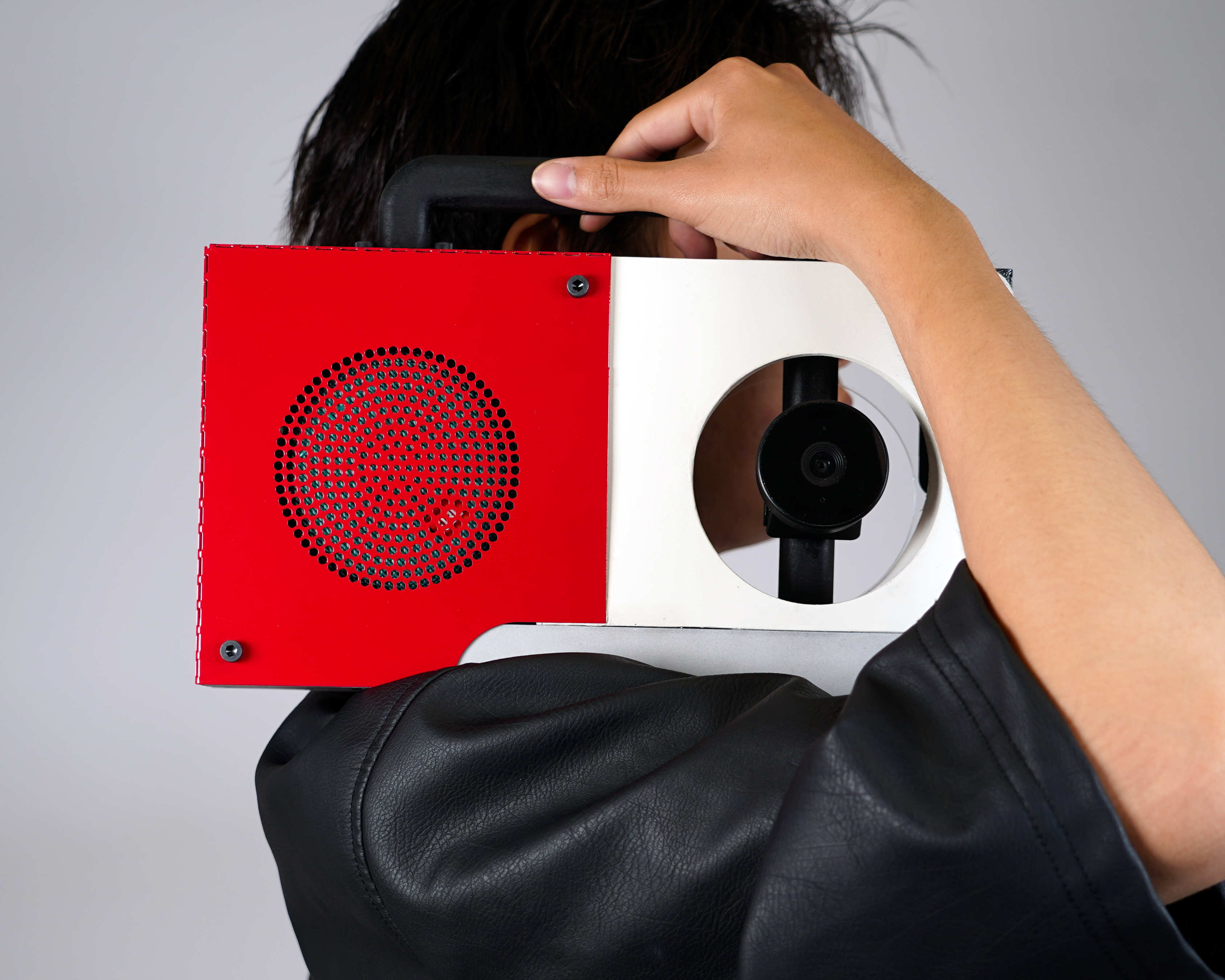
Pilot test feedback
A pilot test of the "Be the Beat" AI boombox revealed promising potential but highlighted areas for improvement. Participants found the device intuitive to activate, though some struggled to understand how movements translated to music generation. While the system impressed users by successfully mapping certain dance styles (e.g., Hip-Hop, House) to fitting music, it was inconsistent in matching genres and failed to reliably recognize nuanced styles like Latin dance. The unpredictability of outputs fostered creative exploration, with dancers discovering new styles and adapting performances, though some desired greater control over genre and tempo selection. Suggested improvements included integrating Spotify for personalized playlists, enhancing movement recognition, and exploring features like emotion-based music selection via facial recognition, offering a more interactive and customizable experience.